Implementation Details
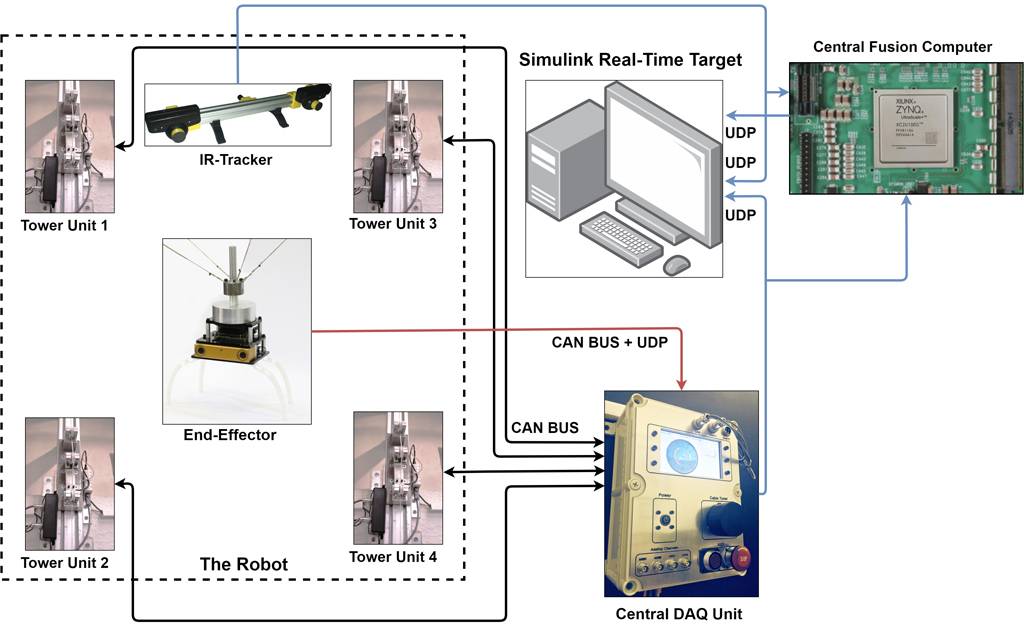
Software and Algorithms:
ARAS-CAM is aimed to be a fast deploy-able robot. This agenda means that the robot should be robust against uncertainties; the instrumentations should be maintainable in the field and, the installation and calibration procedures must be fast and straightforward. As such, We, in the ARAS PACR group, are investigating novel and efficient algorithms to realize this goal.
State Estimation:
The first essential requirement for controlling the robot is to know its internal states. For this reason, we investigate classical fusion as well as data-driven AI perception methods to create a robust and accurate state estimation module for the ARAS-CAM robot. This module exploits the data from visual, inertial, and kinematic joint-space sensors and combines them to yield reliable and accurate measurements. As a deploy-able robot, ARAS-CAM should be easy to install and calibrate at new locations. As such, developing effective multi-modal calibration algorithms is a must. Algorithms that can use the information from the multiple sensors within the robot to estimate its kinematic and dynamic parameters.
No matter how accurate the calibration is or how high quality the sensor systems are, there always remains some amount of uncertainty in the system. Uncertainties that can either be due to simplified mathematical models or their parameters. As such, we investigate robust and adaptive control strategies that can tolerate this discrepancy. The robot is comprised of mechanical structures whose movements are sensed through a network of sensors and controlled using servo actuators. These network of sensors and actuators are controlled by a hetrogenous real-time embedded system. Most of these hardware components and modules are designed and implemented in the ARAS PACR lab.
The robot is made up of four tower units, and an end-effector connected to them through four cables. The towers are anchored to the ground, and each contains a 750W AC servo motor and embedded cable force sensors with CAN-BUS synchronized amplifiers.
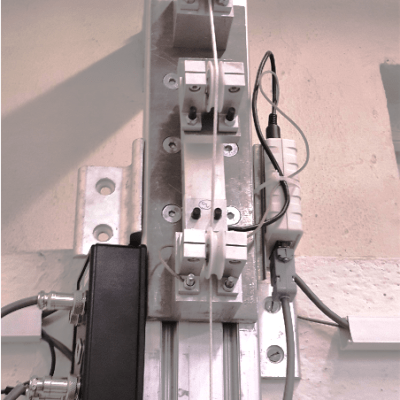
The end-effector is the ARAS-CAM carries a gimbal camera kit and is equipped with two vision sensors and an IMU for estimating the end-effector’s position. One of the vision sensors is facing downwards while a stereo camera observes the front environments. In addition to these sensors, an Nvidia Jetson computer is installed on the end-effector. This computer is responsible for executing the vision algorithms and sending the results to the central DAQ system.
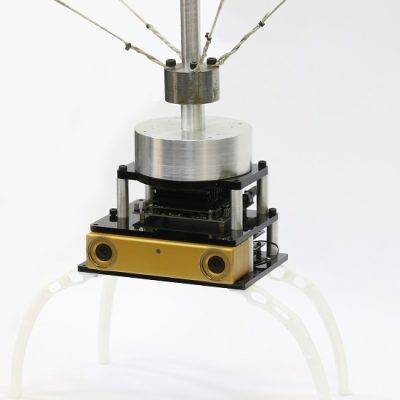
To accurately measure the end-effector’s position, we have developed an infrared tracker system using two high-speed cameras. The measurements form this system is used as ground truth for evaluating the control and state estimation algorithms. We have provided a tutorial on how to build this system Here.
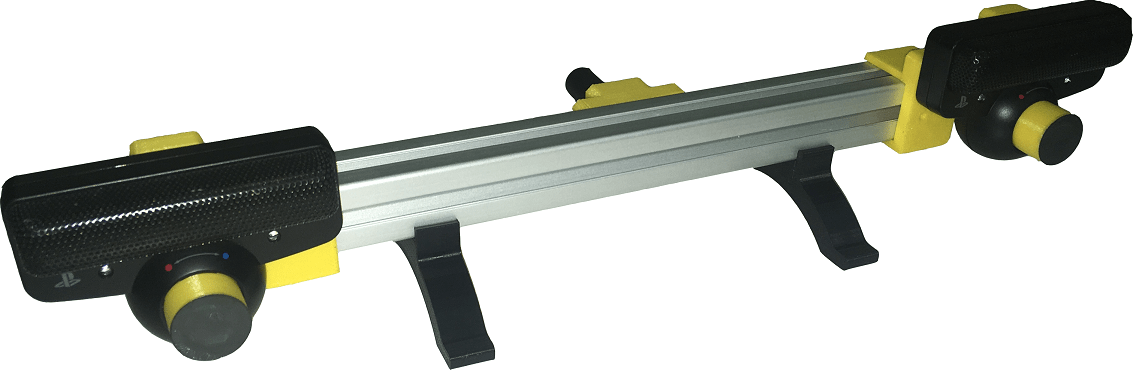
The control and estimation algorithms in the robot should be executed at a high rate and with low latency. Since the processing loads in the robot algorithms have different natures in terms of real-time constraints and processing intensity, their integration into the robot and their implementation require an embedded processor that can offer proper resources for all of these processes. For this reason, we have used the Xilinx Zynq family of SOCs to create our central embedded computer. In addition to this platform, the visual perception and AI inference tasks are delegated to the Jetson computer installed on the end-effector. Parallel to these systems, we also use the Matlab Simulink Real-Time target to accelerate the development process.
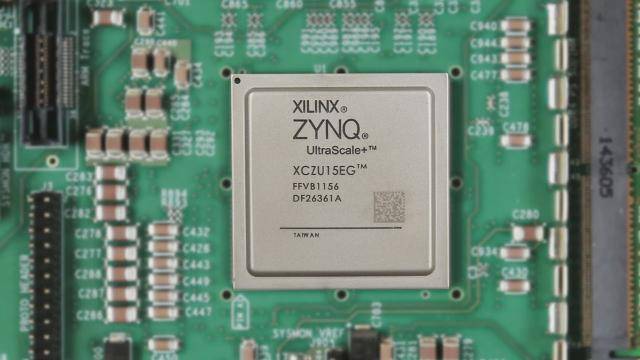
All the data from the sensors within the robot and calculated control signals are managed by a central data acquisition system through UDP and CAN-BUS connections. This system is designed and built based on an ARM STM32F7 microcontroller and an embedded Linux computer for GUI management.
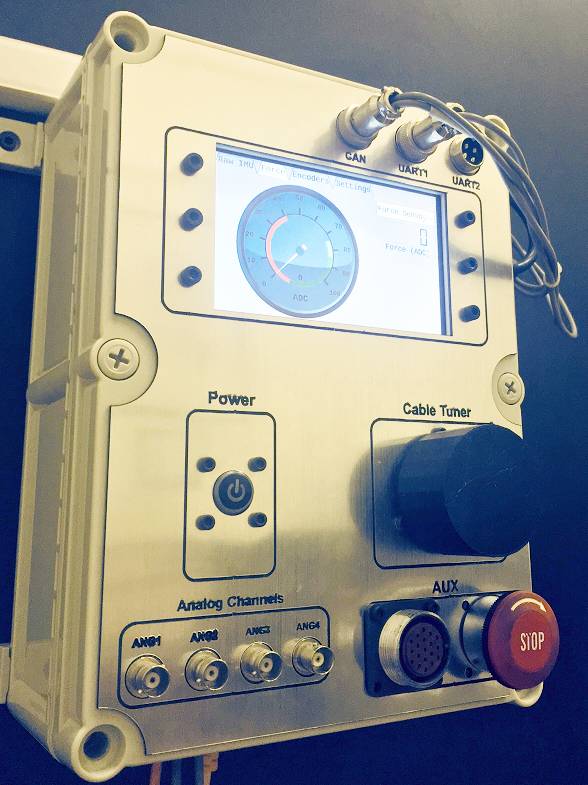